The Complexities of AI for Project Scheduling
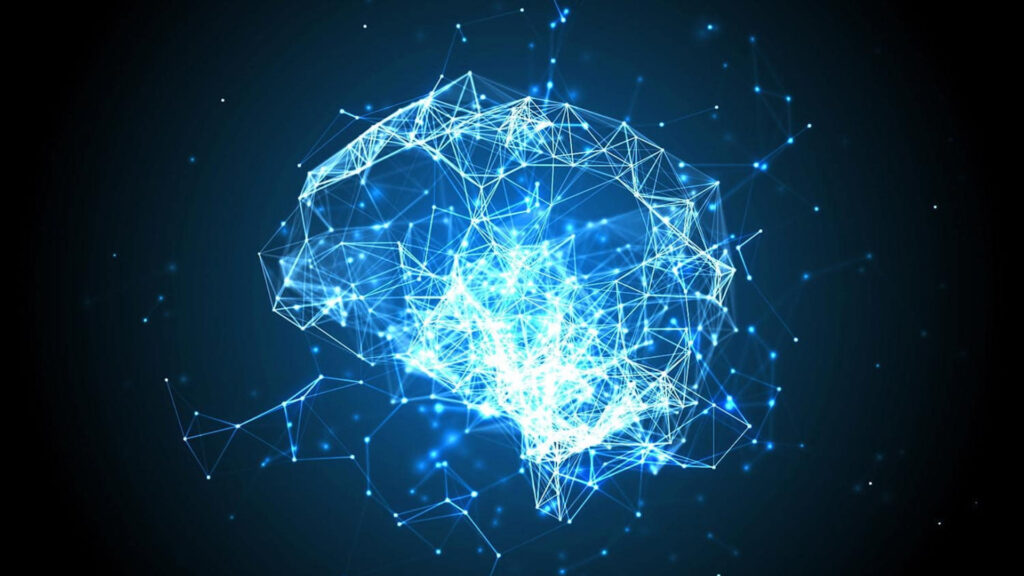
Project scheduling has long been one of the cornerstone processes in project management. It dictates how tasks are sequenced, resources allocated, and milestones established, ensuring projects stay on track and stakeholders remain informed. With the rapid advancements in Artificial Intelligence (AI), it’s only natural to envision AI-driven tools revolutionizing this process. While AI promises to enhance scheduling with predictive analytics, automation, and optimization, it also introduces several complexities.
- Data Quality & Quantity: The efficacy of AI is heavily reliant on the quality and quantity of data fed into it. For project scheduling, this would mean historical project data, task durations, resource availabilities, dependencies, and more. Poor or insufficient data can skew AI predictions, leading to inefficient schedules.
- Dynamic Project Environments: Unlike structured tasks that AI excels at, projects often operate in dynamic environments. Resource availability, project priorities, or stakeholder requirements can change. AI algorithms would need to be adept at handling these uncertainties, making them more complex than traditional deterministic algorithms.
- Interpretability: One of the challenges with sophisticated AI models, particularly deep learning, is their lack of transparency. When an AI suggests an optimized schedule, project managers might find it challenging to understand the reasoning behind certain decisions. Without this understanding, gaining stakeholder buy-in or even trusting the AI’s suggestions becomes difficult.
- Over-optimization: There’s a risk with AI-driven scheduling to over-optimize. While a super-efficient schedule might look impressive on paper, it might not be practical. Buffer times, for instance, are essential in project scheduling to account for unforeseen delays. An AI striving for maximum efficiency might trim these buffers too much, leaving no room for contingencies.
- Integration with Existing Systems: Many organizations use established Project Management Information Systems (PMIS) or Enterprise Resource Planning (ERP) tools. Integrating AI solutions with these legacy systems can be complex, requiring significant effort and potentially altering existing workflows.
- Training and Onboarding: For organizations to truly benefit from AI-driven project scheduling, both project managers and team members need to understand its capabilities and limitations. This might involve extensive training and a cultural shift towards trusting machine-generated insights.
- Ethical Considerations: As with any AI application, there’s a need to ensure that the algorithms are unbiased. If past project data contains biases (e.g., always assigning specific tasks to certain demographics), the AI might perpetuate these biases in future schedules.
- Cost Implications:
Implementing AI solutions might involve significant upfront costs, including acquiring the technology, integration, and training. It’s essential to weigh these against the potential long-term benefits.
While the application of AI in project scheduling offers immense potential benefits like efficiency, accuracy, and foresight, it isn’t a silver bullet. The complexities associated with its deployment highlight the importance of a balanced approach, combining human expertise with AI insights. As the technology evolves, and organizations become more accustomed to its nuances, AI will undoubtedly play a more substantial role in shaping the future of project scheduling. But for now, a collaborative approach, valuing both human intuition and machine intelligence, seems to be the most pragmatic way forward.